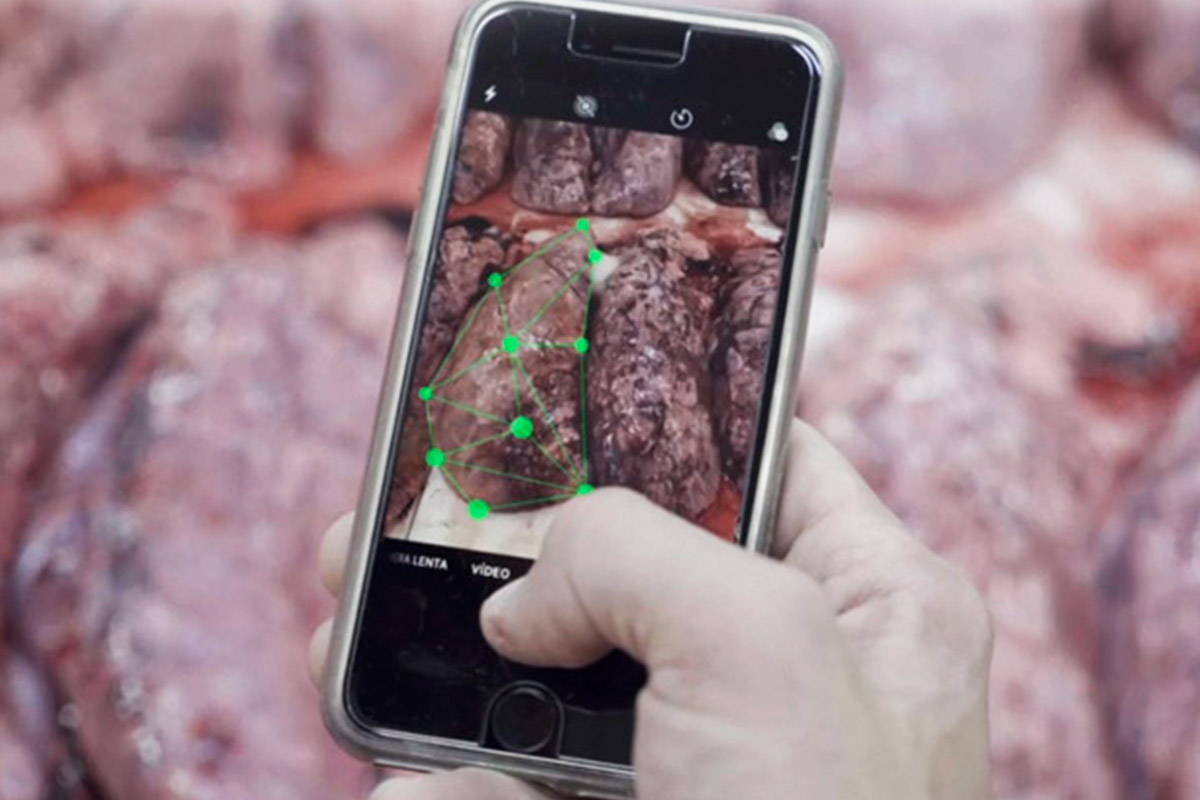
The assessment of pulmonary lesions compatible with Enzootic Pneumonia at the slaughterhouse is one of the techniques of reference for assessing the incidence of this disease in porcine farms and it is the first technique for assessing the effectiveness of vaccines against Mycoplasma hyopneumonia (Mhyo).
Introduction
Until now, monitoring the degree of pulmonary involvement caused by Mhyo required, first of all, highly skilled technicians to take care of the pulmonary score at the slaughterhouses.
These technicians had to be present at the slaughter line, with the accompanying restrictions, in order to be able to manually and individually check every single lung and keep the score on paper or on a tablet computer.
The process is undoubtedly slow and time-consuming and, even if carried out by skilled personnel, the disparity of the pulmonary assessments carried out by different technicians reveals this is a subjective process, based on the human factor.
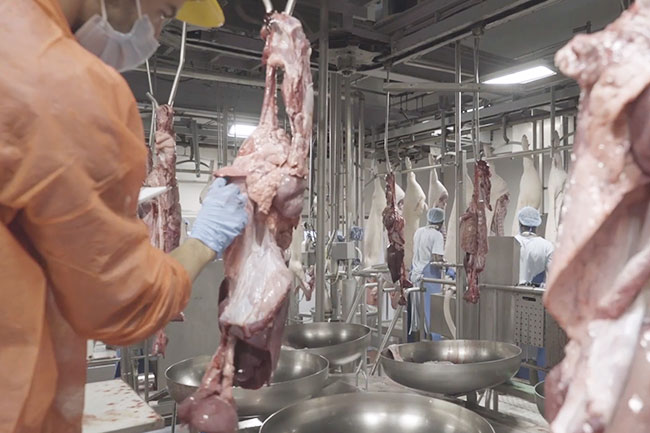
As for Atrophic Rhinitis, the situation is very similar because skilled technicians have to go to the slaughterhouse, separate the heads, cut the muzzle and assess each and every animal individually, keeping the score of the spiral lamellas and the septum on paper or on a tablet. Again, a subjective and time-consuming process.
Artificial intelligence (AI) is defined as the ability of a machine to present the same capacities as human beings.
AI allows technological systems to perceive their environment, relate to it, solve problems and work with a specific purpose. The machine receives data (already prepared or collected using its own sensors, for instance, a camera), processes and responds to it.
AI may seem a futuristic thing and even spook some people, but the truth is AI is already present in our daily life: when we use our GPS to find the best way back home, when we have a robot clean our homes or when we search for anything on the Internet. AI has many applications, also in human and veterinary medicine, which can improve the quality of life of ordinary people and professionals in every sector, as well as the efficiency of processes related with work routines in various sectors.
Monitoring lungs or nasal turbinates is part of the vet’s routine health control of their pig farms. This monitoring work is tedious, complicated and completely subjective, which is why it is a clear candidate for automation using AI technology.
Given our constant commitment to innovation, HIPRA decided to go one step forward and develop a new, fully automated diagnostic system, which allows to simplify, objectify, and facilitate the whole process of slaughterhouse assessment of Mhyo-compatible lesions in lungs and of Atrophic Rhinitis in nasal turbinates.
Artificial Intelligence Diagnos (AI Diagnos) is a smart system capable of keeping the score of lesions individually and objectively based on images of lungs and nasal turbinates.
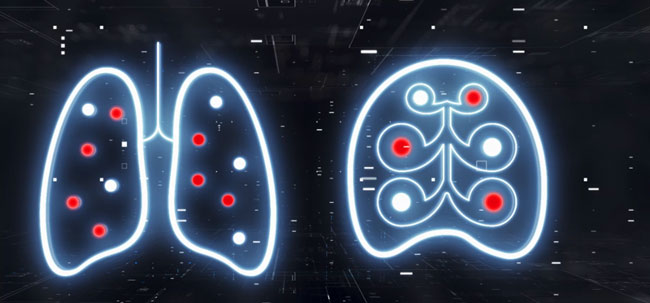
The following article describes the main features of this new service provided by HIPRA: Operation, detection and classification process, output of results and practical applications.
Operation: Detection and classification
Definition of the image detection and classification processes
This section explains how the algorithms detect the various parts of an image and how they are classified, taking into account the two cases developed: Rhinitis and Mycoplasma.
The general detection and classification process is as follows:
1. Firstly, a “filter” (detector of focus) is applied to the image with the purpose of detecting the pig snout (for Rhinitis) or the lung (for Mycoplasma). Once this is achieved, the resulting image is saved.
2. The processed image goes through a detector of areas of interest which identifies each of the target sections for each disease (for instance, septum and turbinates for Rhinitis or the various pulmonary lobes for Enzootic Pneumonia).
3. After these detections, a new “filter” is applied which checks their position within the image in order to rectify potential detection errors.
4. When the sections have been detected, each of them is cut out and saved separately keeping their relationship with the initial image (the image resulting from point 1).
5. Each section goes through a new “filter” (classifier) which is trained for scoring the particular lesion grade.
6. Finally, the total involvement grade is calculated and this information is sent back together with the prediction of all the sections.
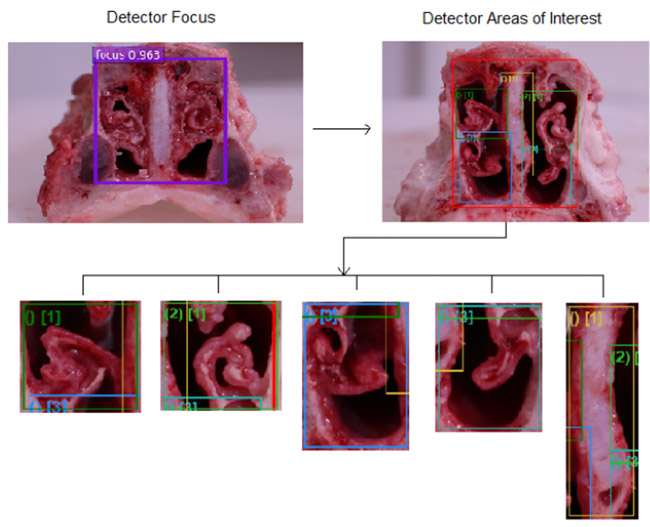
Image 1 – Processing of the Rhinitis model.
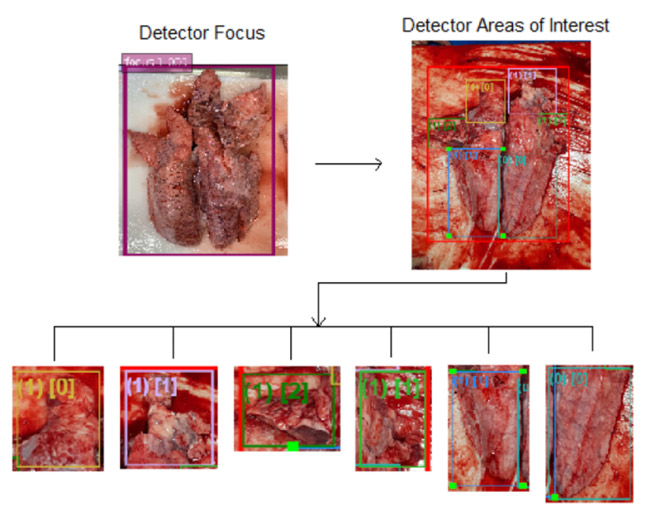
Image 2 – Processing of the Mycoplasma model.
Detection
The detection of objects is a smart image recognition technique which allows to identify and locate objects within an image. AI DIAGNOS has two detectors per model: the detector of focus, which identifies the lung or the nasal septum, and the detector of areas of interest, which identifies the various areas of the lung or the nasal septum.
Each detector needs to be trained in image recognition in order to find the focus or the areas of interest; this is done in two steps:
1. Configuration of the training parameters, where we indicate the number of training cycles and the number of images to be introduced in the network for training.
2. Addition and linkage of images to the system so that the latter can use this data.
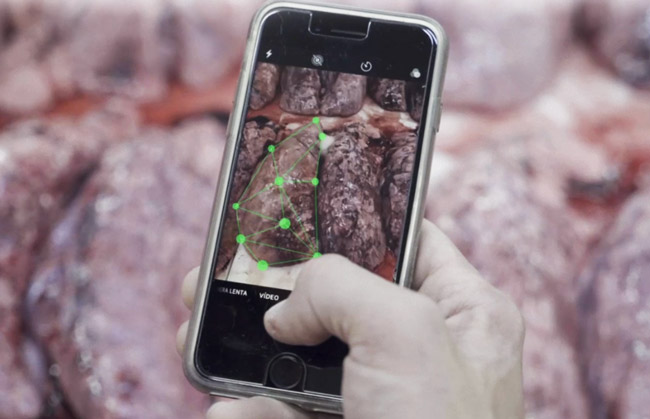
Classification
We have the following sections for each case developed:
· Rhinitis: right-hand dorsal turbinate, left-hand dorsal turbinate, right-hand ventral turbinate, left-hand ventral turbinate and septum.
· Mycoplasma: right-hand apical lobe, left-hand apical lobe, right-hand cardiac lobe, left-hand cardiac lobe, right-hand diaphragmatic lobe and left-hand diaphragmatic lobe.
In order to create our models, we had to train the system to be capable of classifying the lesion grade of the various areas of interest, according to the set of images previously analysed by the same system during training. The model takes each and every image for analysis and shows the lesion grade for each section.
In order to train each model and make it learn the proper assessments, we have to specify:
· The image repository.
· The image transformation instructions, i.e., whether the images will need to be rotated or cleaned of blood or if any other factors which may affect the proper classification have to be taken into account.
The system has been trained using over 11,000 images which have been evaluated by the system and corrected for learning by a number of experts in Mhyo from all over the world, so the system is thus validated.
The result of this validation was that the system currently has more than 85% direct accuracy by system-evaluator, which means that the system and the evaluator have both given the same score, and more than 96% with an error per lobe rate of ±1.
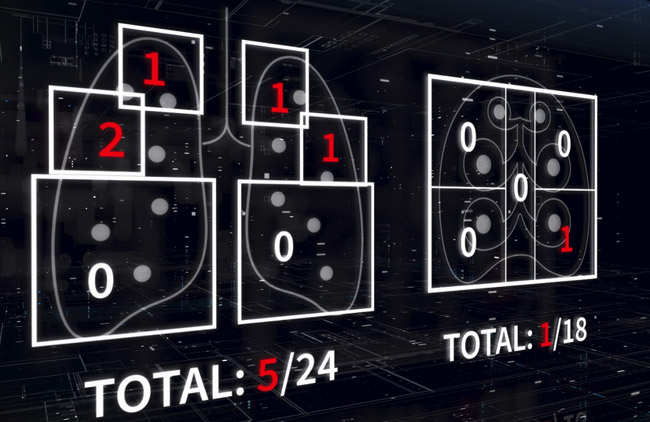
Practical example
AI Diagnos report for a case of Enzootic Pneumonia
In order to start an AI Diagnos analysis, the first thing to do is selecting the company where the evaluation will take place, then the breeding farm (sows) and the growth (fattening) farm have to be introduced and the pathology for analysis has to be specified (Enzootic Pneumonia or Atrophic Rhinitis) and finally the images (lungs or nasal turbinates) have to be uploaded, always in the vertical position and with the pulmonary lobes or turbinates clearly visible.
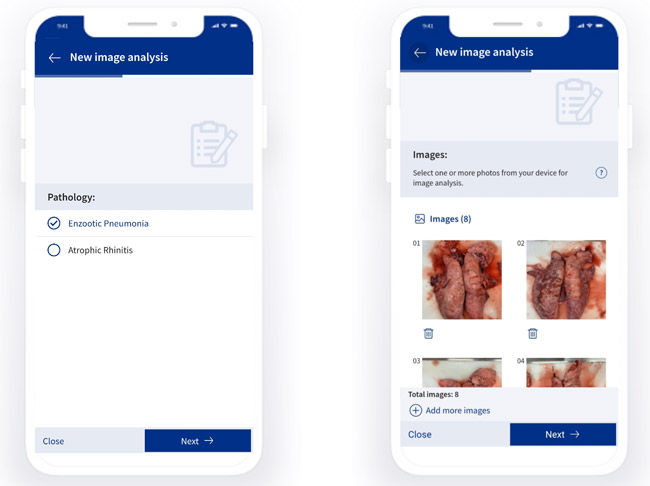
After that, the system starts the analysis, which can take a few minutes depending on the number of images, and then the following report is automatically generated:
First, the lungs are classified according to the lesion grade:
Grade 0: 0% lesion
Grade 1: 0.1-11% lesion
Grade 2: 11.1-21% lesion
Grade 3: 21.1-31% lesion
Grade 4: 31.1-41% lesion
Grade 5: 41.1-51% lesion
Grade 6: 51.1-100% lesion
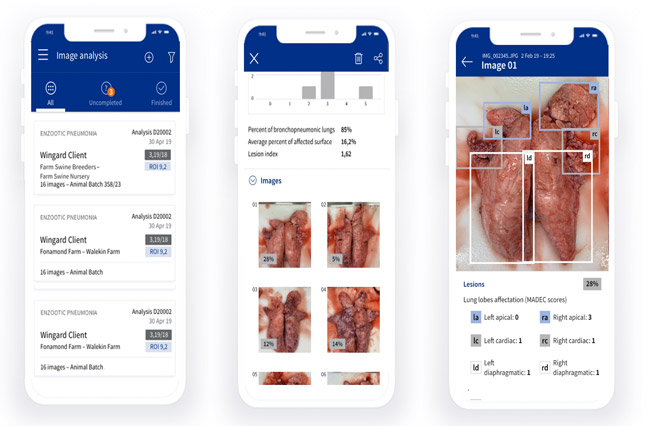
Also, various parameters are analysed, such as:
Disease rate: average lesion grade of all lungs.
Incidence: percentage of lungs with lesions.
Average surface of lungs with lesions: average surface of all lungs with lesions.
Lesion rate: average lesion grade of all lungs affected.
In addition, the system carries out a Return Of Investment (ROI) assessment according to the percentage of animals per lesion grade, because there is a direct correlation between the lesion grade, growth reduction and a conversion increase, which also means extra feed consumption1.
The system allows to download a report in PDF format which contains all the information and can be easily shared.
Conclusions
Artificial Intelligence Diagnos is a great breakthrough for the swine industry, because it is a new system to evaluate lesions caused by Enzootic Pneumonia and Atrophic Rhinitis with has very clear advantages:
· Automated: it only requires taking photos and upload them in the system, so we do not need to send specialised technicians to the slaughters, and we also save time in the individual assessments.
· Reliable: it is a fully objective process, where the subjectivity of the evaluators is eliminated, and the image are always evaluated according to the same set of criteria. In addition to this, as we mentioned before, the system has been trained with more than 11,000 images validated by the main specialists in Mhyo from all over the world.
· Simple: it is a very user-friendly system, where only the farm data has to be completed and the images of the lungs or nasal turbinates’ have to be added for the system to perform the evaluation automatically. In addition, the system generates a report automatically to make interpretation easier for the user.
REFERENCES
1- Straw BE, Tuovinen VK, Bigras-Poulin M (1989) Estimation of the cost of pneumonia in swine herds. Journal of the American Veterinary Medical Association, 195, 1702-1706.